5 stages for implementing HR Analytics: From conception to delivery
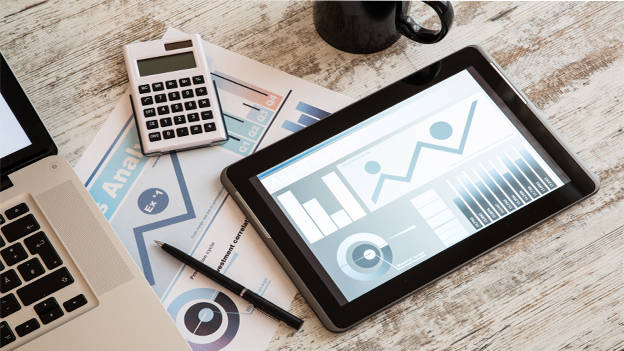
HR Analytics system should have the capability of deeply mining data, learning patterns from the past, and predict future outcomes with some level of accuracy
An HR Analytics system should be in the build-validate mode in its development stage
Today an increasing number of organizations are turning to HR Analytics for decrypting exponentially voluminous data, in a bid to make well-informed HR and workforce decisions.
However, the thought of every HR Analytics system implementation triggers the playback of well-known London Tube announcement in our heads - “Mind the gap”.
At a very high level, HR Analytics implementation translates to:
Disappointment = Expectations – Outcome
Disappointment then, is the positive gap between expectations and outcome. If the outcome surpasses what was expected, then it qualifies as delight, and conversely, when the outcome fails to meet expectations, it qualifies as a failure or a disaster. Hence, what is important is the fact that notwithstanding the desired outcome, building the right expectations is the key to deliver delight. This is exactly what is important in the context of building an HR Analytics system that has its own share of problems. First, Analytics in itself is a fledgling field – everyone either wants to do it, or is trying it but often does not understand how to do it, given the complexity. Second, Human Resources is not a business function like Finance where processes are defined, can be quantified and a number of objective decisions can be taken. For example, Human Resources does not have an equivalent of FP&A (Financial Planning & Analysis), which has existed in most finance organizations for ages. Human Resources has a large amount of qualitative aspects, and not all of it can be quantified or assigned a numeric value, which makes it a very tough case for application of Analytics techniques.
It’s all about setting the right expectations
Let’s look at each of the phases of system delivery lifecycle in context of managing expectations.
Conceive
First thing first. Don’t think of HR Analytics system the same way as your HRMS system. Analytics systems are not transactional systems and are therefore, easy to build but then simply left unused. These systems don’t stop the business from happening. The employee lifecycle will continue without interruption even if the Analytics engine kept getting rusted. Therefore, it is important for the Analytics system to be conceived in a manner that it is used for the purposes it is built for.
Trimester one - What do you wish to achieve
Before we get to what can a good Analytics system do, let’s eliminate the broad aspects of what it shouldn’t do. There are 3 things, typically referred to as the three Bs, which should not be done. These are:
- Basic metrics reporting – the HR Analytics system should not be another Business Intelligence/Dashboard system that churns out volumes or different types of reports.
- Benchmarking – the Analytics system should not be targeted to be a benchmarking system against internal or external metrics.
- Badly designed correlations/statistical analysis – the system should not be used to derive useless and redundant correlations like increasing number of hiring managers also increase the staff turnover or not.
A good HR Analytics system should have the following attributes:
- Story-telling – the Analytics system uses various techniques to wrangle the data, generate insights and recommend or predict business outcomes, all of which can be stitched together to tell compelling stories. These are stories that use hard facts and numbers and enable people to see different scenarios in the future and take decisions accordingly.
- Prediction – the Analytics system should aim to predict and prescribe, rather than simply describe and diagnose. It should simulate future scenarios and predict the chance of a business outcome going in a certain direction.
- Evolving – if an Analytics system is being built with scenarios which will remain constant for next 5 years, it is better to shelve the system. The analytical scenarios are generally dynamic and will change with the state of the enterprise. The Analytics system should hence be a “self-learning” machine that continuously learns from data fed into it and how it is performing to fine-tune its future performance and increase accuracy. It should be a great simulator where people can change different parameters of the business and see what the predicted outcomes could be with a certain level of accuracy.
In summary, an HR Analytics system needs to be a great blend of analysis and synthesis. It should have the capability of mining the data deeply, learning trend patterns from the past, and tell us with some level of accuracy what the future outcome might be. The intention of such an Analytics system is to generate actionable and data-driven insights that make decision-making simpler and accurate.
Trimester two – Grow/Build
During this phase, particular attention should be paid to develop a system, which will be:
- Relevant
- Usable
- Flexible for future needs
Unlike the HRMS or any other transaction processing system, which follows the conventional method of first freezing the requirements followed by a long period of system build with limited ability to change the system, HR Analytics system should be built with the principle of constant user engagement. It may be a good indicator if the requirements keep evolving (not changing directionally) during the build phase. This will ensure that the users are thinking what they want and the system developed in this manner is more likely to be relevant and usable by the end users.
Trimester three – Nurture/Preparing data
This is possibly the only part of preparedness, which can never by over-emphasized. The single biggest failure point for most Analytics systems is quality and accuracy of data. Since the management attempts to make decisions using an Analytics system, the accuracy and relevance of data is must.
There are just reasons to know that data quality challenges for an Analytics system are likely to be more daunting compared to any other system:
- An analytical system is likely to use data from different data sources, which may not be consistent or easy to combine. This is becoming even more challenging as many organizations have been adopting cloud models for systems like 360 degree feedback, employee engagement initiatives, performance management, etc. With proliferation of multiple cloud service providers, aggregating and combining these disparate data sources is a big challenge.
- A good predictive Analytics system is likely to use data from both structured and unstructureddata formats.
- An analytical system can be as insightful as the depth of historical data. This is harder to achieve as the organizations rebuild/upgrade the transactional system once every five years and as an easier “good” practice, the new systems don’t store historical data due to data quality issues. This challenge becomes multifold if different systems have different historical data depth.
As a thumb rule, one must plan for at least 30 percent of total time and money investment in preparing and cleaning data for an analytical system.
Time to deliver
This phase too is quite different for an Analytics system compared to a transaction system. Unlike your HRMS wherein one needs to deliver a near perfect system in first go, an Analytics system need not do everything on day one. It can be good to roll out if it provides just one or two relevant business insights, provided it has no data accuracy issues. Since most analytical systems are likely to be used by domain experts, fewer users, and often used in assisted-mode, it is alright for these systems to have few loose ends.
Like any Analytics system, an HR Analytics system should be in the build-validate mode in its development stage. Post that, the best approach is to start with a pilot on concrete data to observe how the system is responding and behaving. There should also be a well-defined feedback loop that helps the system learn from its performance and increase accuracy. Such kind of a system is never built once and forgotten – it needs periodic review for both performance and accuracy. The system constantly evolves just like ourselves and the data around us.
Conclusion – “It’s an HR Analytics system”
In conclusion, an HR Analytics system needs to be treated like a baby – whom you love and would like to be your support in future, but it really isn’t self-sufficient yet. It will consume a lot of your time to train it to simply start walking! It will demand more and more time from you once it starts growing and learns from you and the ecosystem around it – there will be a time when it becomes smarter and starts learning independently. However, once it is grown up, intelligent and mature, it is likely to have its own brain to start surprising and delighting you with what it can do.