How worried should we be about AI?
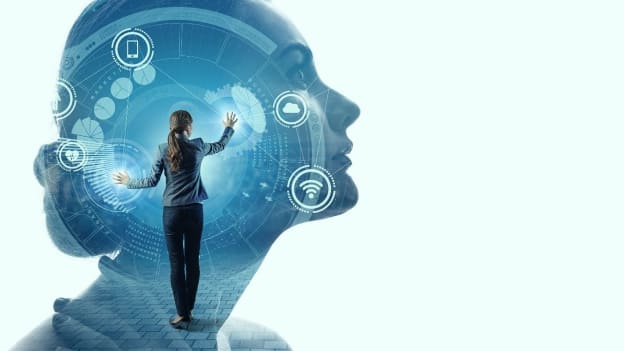
Touch a penny to a live electrical plug: that's what Alexa told a 10-year-old child who asked it for a challenge to do. Amazon's virtual assistant made the headlines in a bad way last month after reportedly scraping this 'challenge' off TikTok and regurgitating it wholesale.
Alexa's gaffe recalls Microsoft's Tay chatbot, which had to be taken down in 2016 after learning to swear and spew extremist ideology from Twitter. Almost six years separate the two incidents, and machine learning should have long since advanced beyond returning such wildly inappropriate responses. It's an important consideration now that AI has become the foundation of multiple workplace tools, especially recruitment and retention. Just in 2018, Amazon had to trash their entire AI hiring tool because it began to unfairly penalise women candidates after studying patterns in the industry – a problem that, like Alexa's potentially deadly recommendation, arose because AI lacks the ability to evaluate data within a wider context.
Why did Alexa come up with such a response, anyway?
“It's very surprising to me that this happened – that they were training their AI on TikTok,” says Sunny Saurabh, co-founder and CEO of Singapore-based Interviewer AI. The issue, he explained, is that because of AI's contextual limitations, it has to be trained very specifically for the function it fulfils, whether education-oriented or entertainment-oriented. A great deal of care must also be taken with natural language processing – the AI's ability to understand what is being said – because human emotion and human reactions may not be appropriately measured or categorised.
What that means is, developers have to be extremely focused and meticulous in their approach. AI cannot be properly trained just by having it observe human interactions, Saurabh says. Doing so is essentially pouring in large quantities of random, uncurated data and letting the model churn out a completely unmoderated response – what's called a black box approach, where there is no real visibility into what goes on inside the algorithm and people only realise something's gone wrong when outrageous results appear.
“To build great AI systems, we want them to learn quickly and for them to learn quickly, AI systems seek big data,” says Anand Bharadwaj, BD Leader at India-based Tiger Analytics.
And raw big data, he points out, is more likely to mirror the worst than the best of human society – meaning that it will contain misinformation, fake news, propaganda, and hate speech, to say the least.
“To solve this, we need data scientists who are good at curating and cleaning the training data with an eye for systemic errors. Many such issues with training data and ML models are easily traceable if AI systems use a White-Box approach for development.”
White box development in AI refers to a consistent, easily interpreted model where results can be clearly understood by a human observer.
Less of a tech issue, more of a people and ethics issue
Bharadwaj puts it down to a bad disconnect between developers and end users, a tendency for tech folks to be highly siloed and overlook customs and cultures while building AI systems.
“Big-Tech companies need more common-sensical people who can work as gatekeepers at the intersection of humanities and technology to solve this problem. Relentless and regular testing, more so by independent third-party companies will also help,” he says.
Saurabh, who has worked with big players including Microsoft and LinkedIn, is more charitable about the Alexa incident: “It's that hunger to exceed which I think is driving them to use TikTok videos as another data source,” he says.
And it's not even about the data per se, he points out: it is fundamentally about ethics, about having the integrity to use professional and reliable sources for datasets even if this is more resource-intensive. It is about curating the process and being aware from the first step that there may be certain biases – such as a company that wants to hire more male candidates, or only wants a certain number of years of experience in a certain field. Amazon's failed recruitment system, for example, did not incorporate any kind of acknowledgement that the system it was learning from is biased to begin with.
“If you use unethical means to develop your model, you will get trash, gibberish,” he warns.
What are some ethical standards to keep an eye out for?
In the training of AI for recruitment, there are a few things to be mindful of. Here are some suggestions by the developers.
Firstly, AI models should be white-box, with the learning process and the decision making process transparent.
Secondly, developers must be very mindful of discrimination and bias – these are all too frequently inherent in data, and curation is critical to ensure the AI doesn't pick up something it shouldn't and run with the bad result.
Thirdly, the model should be periodically audited for bias, fallacies, or other problems with its decision process. Ideally this could be done by independent third parties; if not, at least some internal review should be carried out.
Fourthly, candidates' personal data must be protected and not put to uses other than what it was given for, i.e. recruitment for that particular role the candidate had expressed interest in.
Fifthly, AI should be used in a fair and above-board manner. It's one thing to train AI to identify the best candidates from a pool of applicants; it's another thing to have AI scrape the resumes of competing companies' employees to identify talent to poach.