Navigating through the analytics journey
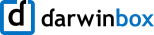
SmarterWorkLife Read similar articles
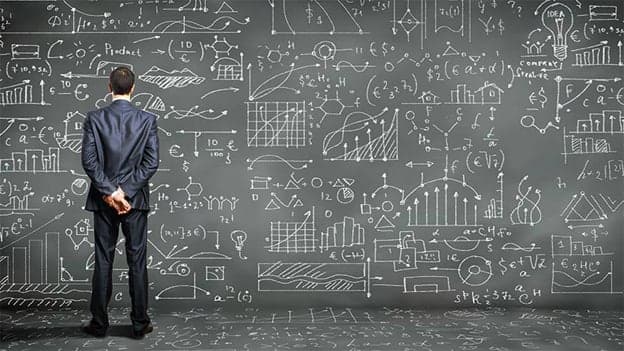
The world of talent is changing dramatically. Thus, the need for establishing linkages and leveraging data in human resources has never been greater.
Data analytics can help organizations ensure hiring and placing of the right candidate for the right job at the right time. However, building data-driven decision making across every dimension of HR – from talent acquisition, performance, and workforce planning to workplace policies, employee engagement, and retention – can be intensely complex and challenging.
To truly provide actionable insights, talent professionals and executives must understand where they currently are on the talent analytics maturity curve, and how to nurture and mature their analytics capabilities. HR should aim to advance from merely describing talent problems to actually prescribing solutions for them.
Here are the four stages of approaching workforce analytics and the value each step provides to your organization:
Stage 1: Automating the complete employee lifecycle
It is crucial to have a single source of truth for data to get captured and consumed authentically, in the right format. So the first step in the HR analytics journey is unifying disparate sources of employee data into a central repository, by way of automating all employee processes across his lifecycle on one single system. This ensures seamless and diligent capture of accurate data. The recommended approach, thus, is to proceed with a central data repository in mind vs multiple sources; and moving to an integrated system that aids by preventing any leakages and inefficiencies. This can be as simple as moving from a pen and paper based system to an automated system for handling exit interviews, clearances and dues during employee separation to begin with.
Stage 2: Having the ability to access data in a flexible yet controlled manner
Access and literacy of technology should never be confined to only some sections of the organisation. Everyone should have access to relevant data under their purview. This data can be pure transactional data that we discussed in Stage 1. However, having the ability to access the required data in any format instead of rigid templates helps in democratising data access and reducing unnecessary dependencies on HR for employee data. “People will not understand how to use it” is nothing but a myth. Thus, democratising and decentralising data is key not just to keep it in multiple lines of sight and control, it widens the demographic of HR owning people analytics, and also the sub-functions within HR that can effectively use and own people analytics. So the missing piece is really not the tech. But the accessibility of data. However, having the ability to maintain strict permission controls and restrictions to ensure people can access only the data they are supposed to is the most critical support required from systems in the view of growing concerns about data privacy.
Stage 3: Gaining insights from processing the transactional data
Processed data can be something as simple as Headcount or Attrition derived from transactional data such as New Joinees and Exits. HR should be able to define their own processed data sets from existing transactional data. This is where data science techniques come in handy based on volume, complexity and aging (real-time vs. some lag) requirement of data. This has the potential to open up enormous and cogent insights for the entire organisation, and contribute meaningfully to business outcomes from things as simple as Leave Utilization and Attendance Patterns to analysing Diversity-Compensation Parity, Performance vs Attrition etc (things that can never be readily available by a human at any given point of time - and take a bunch of time and labour to pull out in silos without going through these stages of maturity in analytics).
Stage 4: Playing with the processed data
This is where organizations should be able to visualize processed datasets on the axes that are relevant for them without any rigidity. It can be a time axis for dataset or organization hierarchy axis for the same or another dataset with an easy to navigate drill down and sum up functionality. Things become even more interesting when there is the ability to visualise multiple disparate datasets together and define your own accesses and parameters. For example, Performance vs. Source of Hiring, Performance vs. Potential vs Comp Positioning etc. Data visualisation presents data in a graphical or pictorial format readily exposing employee behaviors, top talent turnover trends, employee engagement risks, potential dips in productivity, and other such critical talent information. Thus, while the uptake of analytics usage is on the rise, the opportunity lies not in the availability or historical reporting of data but rather in its visibility and ability to interact real-time with it.
Stage 5: Migrating from reactive to predictive
The first 4 stages are about utilizing data reactively for getting insights and aiding future decision making. Constructing organization-specific data models starting with a hypothesis, and using powerful data science techniques will help organizations go to the next level of maturity to predict certain outcomes such as employee attrition, success of a candidate in a role etc. with reasonable accuracy. Machine learning helps to make this process of maturing a model automated as these are dynamic models in any organization, and not static.
When a Regression Analysis is done - you realise that you already have all the data. Age data, demographic data, engagement and effectiveness data. So this step in the journey allows for one to gauge things like success prediction, recruitment channel analysis and so on with much better accuracy. It helps you uncover recommendations and solutions from the data you analyze. So in addition to identifying a pool of employees who are likely to leave over the next year, you are also able to identify actions you can take to address the situation today, well before they have put in their papers. Hence, organizations evolve to predict eventualities and plan ahead for solutions.
The more data you have the more accurate the results will be. Having data on the axises of employee engagement, psychometric analysis will make things more interesting and accurate in the future.
Merits of following the journey vs. buying an off-the-shelf solution:
Unified data with a single source of truth
The process allows you to be able to drill down and see linkages between disjoint sets of data, not only making you appreciate the technology better, but also impacting sounder prediction as opposed to working with data in silos. Experiencing each level of people analytics saves you from disparate employee data sets, strung out across multiple systems and spreadsheets. Thus, the process leads you to have a single source of data.
Seamless data processing
This journey is intuition and common-sense driven - and allows you to understand what data you need to view together. What your hypotheses should be, and what investments you need to make in data science in terms of nuts and bolts and plots and methods. The journey allows you to define, collect, and aggregate people and business data from different sources so you can make fact-based decisions. It aids you identify the most critical data, assess the quality, and help you understand how it can be used.
Interesting correlations and observations
When one journeys with a single system, it’s like raising a child, and teaching it how to emulate the human personality. AI and ML come later, but one must first start here. To mould the raw brain of the technology. The higher the exposure, the deeper the synthesis and the more intelligent the technology. The complete analytics journey helps you ask the right questions, get all the right answers and enable you to make decisions for the business.
Showing you what is most relevant for you
When someone matures in this journey sequentially and naturally, they have far more balanced coverage of the data, thereby enabling the technology to crawl and crunch and churn data from every single nook and cranny. When the tech has the historical pattern and context of what you typically look for from each function - it begins to become more and more intelligent and show you just what you might need.
Right from recruiting to employee training and succession planning, HR analytics plays a key role in aligning human capital practices to broader business objectives. However, despite being on the C-suite agenda for some time now, most organizations are yet to unlock the potential of their people data. The lack of knowledge about analytical methods and tools has been a key barrier for widespread adoption of HR analytics. Hopefully, the framework outlined here can get your organization started on the HR analytics journey.