Bell curve or an 'L' curve? Performance Management once again
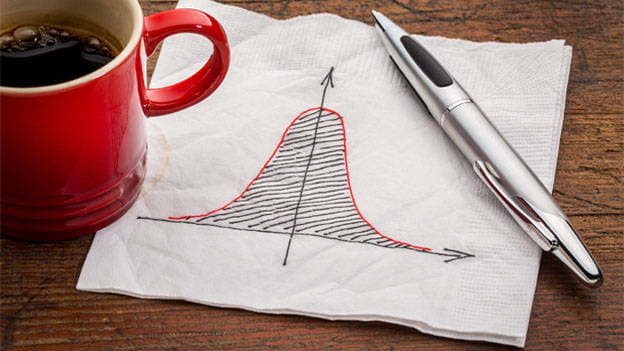
Many organizations have recently moved away from the bell curve distribution of performance ratings. Some of the reasons cited for this change are: it breeds unhealthy competition, it’s the most hated process by managers and employees alike, it promotes political behaviours like schmoozing, and it does not add value to the overall performance management process.
Another reason which deserves attention is the recent research and thinking that shows the increased prevalence of the Power law distribution in performance, instead of the normal / Gaussian distribution a.k.a the bell curve. (The Power law is more commonly known as the Pareto law or the 80/20 rule)
In simple terms, a bell curve distribution for performance means a large chunk of employees perform at or around the mid-point / average level, and a small number of employees are there at both ends of the performance spectrum – performing either outstandingly or poorly.
Whereas, a Power law distribution for performance means a very small percentage of employees contribute a disproportional amount of output. In 80/20 parlance, it means 80 percent of output comes from 20 percent of employees.
Let us take a sales example and understand this better. If the performance of salesmen (selling insurance policies) follows a normal distribution, it will look like figure 1 (bell-shaped) where most of the salesmen perform around the average of 525. And, if it is a power law distribution as shown in Fig. 2 (kind of an L shaped curve), then most of the salesmen are performing below the average of 398, and a few “superstars” towards the tail-end of the horizontal axis are contributing the biggest chunk.
A very long-held assumption in Human Resources is that employee performance follows a normal distribution (bell curve) - an outcome of observing employee performance in the manufacturing industries - the typical assembly line scenario where employee performance was clustered around the average.
An interesting research conducted by Aquinis et al , revisits this assumption of a bell curve. According to their research, individual performance follows a power law distribution, where a small percentage of individuals (superstars) make a disproportionately large contribution to the results. They say that the top quartile of performers contributes about 40-50% of the total results, and a large percentage of individuals lie below the statistical average performance.
At first glance, this is disturbing! Does it mean organizations have got performance management completely wrong till now?
Not necessarily! Keep these 2 things in mind:
1. The word “average” used in their research implies a “statistical average” and not “average” as we mean in daily usage, where it usually implies “commonly seen” or “typical”. In a power law distribution, the variation between the highest performers and average performers is large (refer to Fig.2), therefore a lot of people perform below the statistical average performance level. That does not mean that these people are non-performing, or that they are not adding any value. It simply means that there is a large gap between the highest and the average performance levels.
2. The professions the research looked at were – researchers, entertainers, politicians and athletes. Their research points out that power law distributions are more likely to be found in 21st century professions employing knowledge workers, whereas normal distributions were more likely in the manufacturing era.
Manufacturing jobs typically use manual labour and have infrastructural / technological constraints, which forces performance to remain within the bounds of a normal distribution, and does not let superstars emerge.
Bill Gates gives a good example to understand this better – He says "A great lathe operator commands several times the wage of an average lathe operator, but a great writer of software code is worth 10,000 times the price of an average software writer." Lathe operation is a typical manufacturing era profession, whereas software coding is done by a knowledge worker, and is more of a 21st century profession.
What could have been going wrong?
In cases where organizations forced a normal distribution upon a power law distribution, then the impact of the high performers would have gotten underestimated, and the impact of the lower performers got overestimated. See Fig. 3 below
Why should we be worried about the distribution?
Reward allocation should follow performance distribution. Simply speaking, salary differentials should reflect performance differentials. Using salary differentials as a proxy for performance differentials (assuming power law distributions of performance), we see that in some jobs the performance of high performers has been underestimated. The compensation difference between an outstanding and an average performer even in many 21st century professions goes only upto about 1.5 times. However, we have got it right in some jobs like sales where variable compensation prevails and performance can be clearly measured in numbers. Only a handful of organizations have instituted salary differentials going upto 300% within the same job grade. Organizations that follow a high salary differentiation policy have higher employee engagement.
The problem of measuring performance is actually at the core of the question – Does individual performance follow a normal distribution or a power law distribution? In many jobs it is difficult to measure performance objectively, and subjective measures of performance are used.
How do we correct it?
- Effective objective setting offers a partial solution. Setting SMART (Specific, Measurable, Ambitious, Realistic and Timebound) objectives. Unfortunately, many organizations don’t do a good job of this. If you don’t know exactly what is to be achieved, how will you measure if it has been achieved or not?
- An attempt should be made to differentiate roles where performance follows a power law distribution from roles where performance is more normally distributed. This is not an easy task, and there may not be any exact / scientific method to do it.
Some guidelines for identifying roles where performance may follow a power law distribution are – high complexity roles, no situational constraints (information / technological / geographic / infrastructure etc.), flexible hierarchies, high individual autonomy and less process orientation
- It is possible that within the same organization there are roles which have a bell curve performance distribution, and other roles may have a power law distribution. It is important to recognize this difference and plan reward allocation accordingly
- In a power law distribution there are categories even within the high performers – meaning that in the top 10% of the organization, there is a top 2% whose contribution is much higher than the balance 8%. So, a “finer sifting” needs to be done, and the top 2% need to be looked at differently in terms of career planning and development
- In today’s age and time, the case for variable pay is already strong, and the prevalence of the power law makes it even stronger. Many organizations are still lagging behind in the implementation of variable pay, and are losing out on performance gains. The pay differential (between outstanding and mid-range performers) for roles which have a power law distribution should be higher, than the pay differentials for roles where individual performance is closer to a normal curve. It may be a good idea to start slow on the variable pay vector, and slowly ramp it up.
HR practitioners need to understand performance distribution for different roles within their organizations and plan HR processes (performance management, compensation, career planning, learning & development etc.) accordingly.
A big challenge that power law distributions bring to the fore is the increased perception of unfairness and inequity. There is a human tendency to “keep to the middle”, which the bell curve has been satisfying for very long. However, the reality is painful – that there are elite performers who need to be rewarded accordingly. Organizations which have been “keeping to the middle” will find it very difficult to manage this transition. There is no magic bullet, and the journey will be agonizing. Maintaining a sensitivity to human emotions, while balancing business interests will be a critical skill needed for HR practitioners to make it through.
Reference:
1 The Best and the Rest: Revisiting the Norm of Normality of Individual Performance, O'Boyle, Ernest, Jr.; Aguinis, Herman, Personnel Psychology, v65 n1 p79-119 Spr 2012