Preventing AI Bias: 4 Key approaches for leaders to create fairer systems
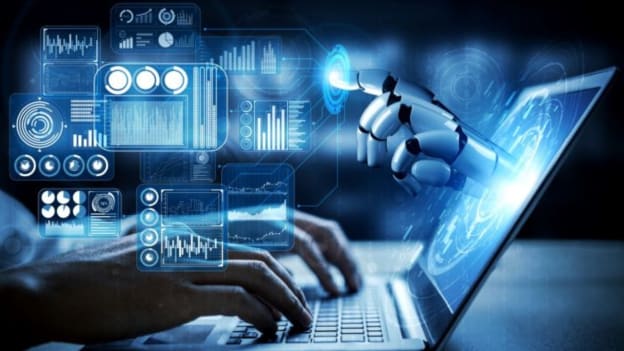
In recent years, Artificial Intelligence (AI) has been the buzzword in the tech world, promising to revolutionise businesses across industries. The Covid-19 pandemic has only accelerated its adoption, pushing companies to seek innovative solutions to enhance productivity, decision-making, and the customer experience.
A PwC study revealed that AI has become a "mainstream technology" at their company, offering tangible benefits that drive growth and efficiency. Similarly, Forrester's survey predicted that almost all companies would be using AI by 2025, indicating a massive shift towards this transformative technology.
However, it's important to recognize that AI is not a standalone solution, but rather a product of human ingenuity and innovation. As such, the technology can be subject to human biases that impact its outcomes and effectiveness. The data and algorithms used to create AI systems are ultimately decided by humans, which can lead to unconscious biases that undermine their potential.
Therefore, it's essential for leaders to adopt a proactive approach and recognise that biased AI is more of a human issue than a technological problem.
Here, we explore the critical importance of addressing biased AI as a human issue, and we provide strategies for leaders to adopt to ensure AI remains a tool for positive change.
Sources of AI biases
There are two main issues that leaders should address: training data (is the data available complete?) and data sampling (is it representative of all people?). AI systems acquire decision-making skills based on the data they are trained on, which might contain biased human judgments or mirror past or societal injustices, even if factors such as gender, race, or sexual orientation are eliminated. For instance, Amazon stopped using a hiring algorithm that was inclined towards candidates who used words such as ‘executed’ or ‘captured’ in their resumes, which were more often found on men's resumes.
AI biases may arise if the sample data contains over- or underrepresented groups. For instance, it was found that AI-based speech recognition technology had higher error rates for individuals with non-native English accents compared to those with native English accents. This bias was linked to the lack of representation of diverse voices in the training data used to develop the technology.
Four ways to handle AI biases
Stay up to-date: Business leaders should stay up to-date in this fast-moving field. They should try to obtain resources/data that provide insights into AI implementation and utilitarian value. It will be important to consider the way an organisation operates. The ways should not disadvantage certain social groups, such as discriminating against individuals based on their race, gender, profession etc.
Transparency: Leaders should maintain transparency with stakeholders to help people understand how AI algorithms create predictions and make decisions. AI is commonly perceived as a "black box," where users can only see the inputs and outputs without any knowledge of the inner workings of the AI. To address this challenge, organisations should strive for explainability so that people can comprehend how AI operates and its potential impact. This could be achieved by comparing results between algorithms and human decision-makers, utilizing "explainability techniques" to determine the reasons behind a model's decision, and running algorithms alongside human decision-makers. If bias is identified, it is not enough to simply modify the algorithm, business leaders must also enhance the human-driven processes that underlie it.
Multidimensional communication: Leaders must incorporate a multidimensional view to establish effective communication. Views from innovators, creators, implementors, and consumers can help leaders mitigate the risk and develop trust in the AI systems so that they don’t drift into biased territory. An effective association between humans and AI can ensure proper human recommendations on AI intervention in organisational processes to embed ethics and eliminate biases.
Collaboration: Leaders should consider adopting a variety of technical tools and operational practices, including internal ‘red teams’ or third-party audits, to establish responsible processes. Such collaborations can enrich training data and increase inclusivity. Lacking extensive testing and diverse teams, unconscious biases can easily enter machine learning models, leading to biased models that AI systems may automate and perpetuate.
AI has many potential benefits for business, the economy, and tackling society’s most pressing social challenges, including the impact of human biases. However, it is only possible if people trust these systems to produce unbiased results. The benefits of AI have the potential to outweigh the risks if they can be addressed constructively. All the leaders and practitioners in the field should collaborate and conduct research to reduce bias in AI for all.