Decoding HR Analytics
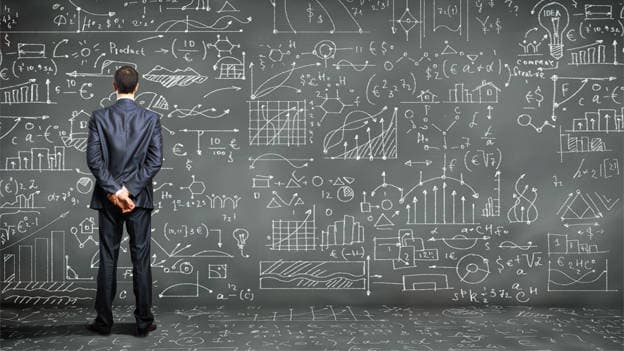
The dynamic and evolving business trends are demanding the HR functions to be bold, courageous, and innovative to be able to add value to their businesses. But how prepared the HR organizations and professionals are to cope with these demands.
Let’s begin by evaluating a few recent facts about HR functions and professionals.
Josh Bersin, Principal and Founder, Bersin by Deloitte, in his recent article spoke in length about the following research findings:
- Among 3,300 HR and business leaders, today the average gives HR a C- in our ability to directly impact the talent challenges in our companies.
- Nearly 1/3 of all new CHROs are from non-HR backgrounds—indicates that CEOs want new thoughts to be brought into the HR function.
- Zenger Folkman research shows that business leaders who are bold in their thinking are 11X more likely to succeed in today's business environment as compared to their counterparts who are practicing good judgment.
As Jay Jamrog, Executive Director of the Human Resource Institute, explains, “Human Resource is crippled by educated incapacity. You're smart, and you know the way you're working today isn't going to hold 10 years from now. But you can't move to that level. You're stuck."
The message is clear. For the HR function to thrive and add value in today's new world of work, we have to be bold in our thinking, bold in our strategies, and bold in the redesign of what we do. We need to ensure that our team is able to innovate and reinvent our HR and talent programs. In addition, we will also need to be courageous and imaginative in your recruiting or management practice. The long-term vision should be to be the most agile HR organization that can proactively align to business context and therefore help win the market we operate in. But the question is how!
As a data-oriented and trained analytics professional, I am inclined to predict using historical data and evidence. But the way technology and markets are evolving; I have begun to doubt the relevance of historical data. No doubt that it can provide insight to what went well and what did not go so well. We can also learn not to repeat the same mistakes. But is it enough? The response is, certainly, not. Nevertheless, the idea is to engage certain practice to explore the unseen for better decision making.
Predictive analytics are a rapidly upcoming trend in HR to explore the future. Predictive analytics is everywhere. It is in essence a technology that uses existing data to forecast individual behavior very specifically. Moneyball—the famous biographical sports drama as rightly unfolds the super power of predictive analytics, features the coach using team analytics to assemble a competitive baseball team that wins 20 consecutive games.
Ironically, even though a lot of people are talking about predictive analytics, hardly any organization is applying it to their workforce. HR’s uptake of analytics is “stuck in neutral”, according to Bill Pelster, principal at Deloitte Consulting. Deloitte’s annual report into HR trends reveals a yawning gap between what HR professionals and the business believe is important and the capabilities of HR to deliver what’s required. This capability shortfall is seen across the board, from the top concerns of engagement, leadership and learning, to key HR technology issues such as analytics. The report revealed that only 8% of respondents believed their organization was strong in analytics, even though 75% saw it as an important issue.
At Genpact, we are on an exciting journey of leveraging science to understand how we engage with our workforce, learn faster and move boldly on data oriented insights. Truly, it is a journey not an end product for us. It is about commitment to key business outcomes over a period of time. It is not a project in silo. The initial struggle was to set up a vision and a mission for this center of excellence. We connected with our CHROs and our key business leaders to find out the challenges they face and discussed the possible scientific approach that we can engage to solve these challenges over time. Let me reiterate here, the purpose is not to solve these issues for reporting needs and insights. We are solving for difficult mathematical problems that have business impact if we get to the solutions.
There are many challenges in this journey that we have embarked. One of the key challenges that we faced in our first 100 days of this journey was to separate the reporting needs from hypothesis testing. A lot of our colleagues wanted to create a good report with good insights and confused it with HR Analytics. A careful analysis is important in such situations. Otherwise, we may quickly degenerate our focus into reporting and visualization of HR data.
The second big challenge of this journey is the data itself. Clean, point in time data is rare in HR systems across the world. We have legacy data dictionaries and legacy source systems with limited smart drop-downs that we need to solve for. Any predictive analytics project across any functional area is a function of data quality and thereafter, the quality of models is built around it. The challenge is that everyone is excited about building the model but no one is willing to clean or treat the data first. We realized very quickly that some of the interesting variables (factors) didn’t exist in our systems and a few of them were not necessarily in the right format (continuous vs discrete). We had to start with setting up the correct measurement system for all the key variables that needed to feed any problem statement. If we are solving for retention, then we need to know when and how someone gets recognized (yes, both when and how are important here!). Likewise, we had to do a grunt work of cleaning up a few data files to ensure that we are building a model, which represents the reality as close as possible and can test a hypothesis accurately. Otherwise, we know that trash in will get us trash out! So, first, we need to address the data quality issue. At the same time, it is okay to live with some level of uncertainty with data. We can’t get paranoid about it. We also understand that we need not get too obsessed with data cleansing either. The fact is data is never going to be sparkling clean. Instead, we focus on the data that we have in hand to get better information out of it, while continuously working to improve it as well.
The third interesting challenge on this journey is capability building around analytics. A recent People Matters survey answered it well, that a lot of organizations are experimenting with a mix of external sourced capability as well as internally built-out capability. We too tried a hybrid model to start off on this journey, it has only been 6-8 months for us on this exciting journey, but we have learnt a lot of interesting lessons for the future. The learning is we need to have enough knowledge of our systems and data patterns to help our vendors and partners make better models for us. We got the right people on our team who knew data and HR well enough to provide the right level of directional support to our analytics experts. Eventually, we have to solve a business problem and not a math problem. Hence, we need that context and validation during this process of prediction and insight generation. We need to get the right HR people with the flair for numbers and the right partners onboard for this long journey.
The fourth interesting challenge that we often face is related to the Personal Identifiable Information (PII) of our employees. The IT Act states that disclosure of sensitive personal data by a corporate body with any third-party shall require prior permission from the provider of such information, unless such disclosure has been agreed to in the contract between the corporate and the provider of information, or where the disclosure is necessary for compliance of a legal obligation. We had to be compliant to use information around gender, age, salary information, marital status, and health issues etc. as we built our analysis with our vendor partners. The data needed to be masked if it had to go out of our systems to be used by a third-party. We had to run into clearance internally with our legal teams to ensure that we are compliant with law and safeguard our employee data at all cost. The learning was that we need to account for such challenges proactively to avoid challenges at a later stage.
The fifth and the last interesting challenge is to set the right expectations about the performance of the model. A statistical model is as good as its data attributes, techniques, and the quality of data that is fed into it. A model development process is an iterative process that improvises over time. We might have trashed 50 odd models to get to the 51st that we liked. So, patience and perseverance is the key to short-term success as well as long-term view of the model. It is advisable to set bridge goals for models to achieve but also have a vision statement for the team to rally around.
These models are incredibly valuable. But as the sports drama flick, Moneyball, points out very clearly, "having the answer does not make it happen." So, having the model is not enough. That is the next challenge for me and our team. The machine will get smarter with better data and validation process but the biggest test will be to make the business smarter with greater insights and more powerful proactive action.
Hope, this provides a better insight into the practical challenges we face while implementing analytics in our HR functions. Let’s keep sharing our stories so that we all learn from each other’s experiences and apply to address our practical problems. As it is rightly said--it's not the findings that make your company unique, it's what you do about them.