Impact of Artificial Intelligence on strategic HR decision making
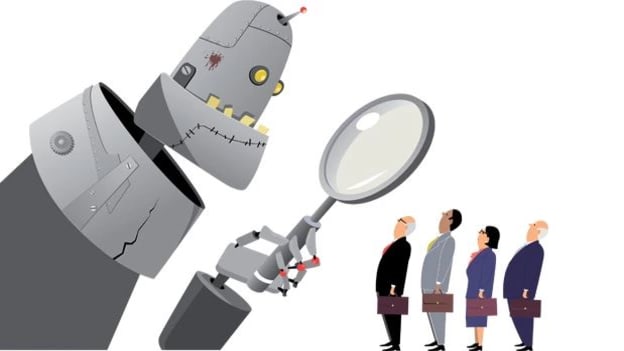
Various studies have time and again thrown up a startling fact—cutting across business segments and verticals in Corporate - financial capital and economic assets are available in reasonable abundance to most businesses, but their monetization or value unlocking has remained a sore point. To make good on this, there is a need for the Human Resources function to step up to the plate and be the differentiator that delivers value to stakeholders.
But herein lies an oxymoron—in most businesses, the HR Function is seen as an administrative and enabling function, rather than a strategic business function. The key reason is the HR teams’ inability to link its initiatives with business strategy or a business problem, and quantify its impact on business. In conclusion, Artificial Intelligence (AI) will help the function take strategic HR decisions that impact the very business and help quantify such impact.
Let us examine how AI will impact HR decision-making in different parts of the employee lifecycle:
Manpower Planning and Productivity Management:
If a CHRO is asked how he measures the productivity of the employees, the usual answer is “based on manager’s feedback” or “based on internal customer feedback”.
Going forward, all the individuals in the team may be asked to list the tasks they accomplished each week, and/or the activities they performed for these achievements, and the time taken for each activity. These may then be analyzed using AI to arrive at the effectiveness and efficiency of individuals, as well as optimum resourcing of each function.
The Sales Function, for instance, is usually evaluated on achievement vis-à-vis targets or historical achievements. Very few companies consider missed opportunities and optimal revenue extraction from the market, clients or the distribution system. This calls for a complex analysis of the market, the competition, and the marketshare of clients’ wallet and the share of distributors’ business, coupled with sales professionals’ skills, effort and attitude. If the data on market, clients and distribution—extracted from the CRM and accounting system, the effort from the sales log and from geo-position tracking—is analyzed together with skills and aptitude from the competency assessment tools. This analysis would make it possible to arrive at optimal targets, along with the target markets, clients or distributors to focus on. The efficacy of sales professionals, along with an ideal profile and optimal staffing, can also thus be arrived at.
A similar analysis can be carried out for ‘Operations’ and ‘Customer Service’ personnel, which would be simpler as the uncertainties in these functions are lower. Having determined the manpower plan and ideal profile for different roles, effective hiring decisions need to be made.
Recruitment:
The maximum time and attention of senior colleagues is invested in leadership hiring, which should ensure a good strategy, planning and direction for the business. However, the execution of the set strategy is driven by front-line sales, operations and customer service personnel, who are recruited by front-line managers and junior HR colleagues, who may not be adequately trained and experienced in structured interviewing, or Behavioral Event Interviewing (BEI), to assess the functional and behavioral competencies and test the claims of achievement in previous job roles.
Research done by Jason Dana, an assistant Professor at the Yale School of Management, and his team, reveals this: “The problem with (unstructured personal) interviews is worse than irrelevance. They can be harmful; undercutting the impact of other or valuable information about the interviewee.”
While it may be difficult to train front-line managers on BEI, especially with high attrition in the segment, and monitor execution; it may be easier to train the machine on BEI and ensure impeccable execution, leading to better hiring decisions that would predict performance significantly better than that predicted by present hiring practices. This could be coupled with the data from online tests on functional competencies, behavioral competencies and personality tools. AI could be used to find patterns between the outputs of these tools and that of BEI on the one hand, and performance of people on the other. While machine learning will help make superior hiring decisions, it will also eliminate the need of front-line HRBPs and free up front-line managers to focus on their given job roles.
Gradually, hiring decisions for middle management and senior management may also be made or at least supplemented by AI. Such decisions will be significantly superior to the decisions made by Managers in the present form. Thus, HR could focus on hiring of CXOs and certain unique roles that do not lend themselves to AI, especially as there are no patterns for want of numbers.
Performance Management:
After hiring, the next significant decision is that of evaluating performance. The usage of AI in goal-setting has been discussed in the ‘Manpower Planning’ section. Let us now understand how AI will impact feedback and appraisal, which is the biggest challenge in any performance management system. Managers shy away from giving hard feedback as it calls for the science of significant evidence, as well as training on the art of giving feedback. Training a large number of managers, monitoring execution and providing feedback to managers on how such conversations could be more constructive and how to avoid destructive conversations is a herculean task for any large organization. Such feedback can be conducted on an AI-based video platform, which would record conversations. The machine will also collect performance data as well as lost opportunity data to provide evidence for feedback. After the feedback session, it will collect post-feedback performance data to create patterns of good and not so good feedback based on the performance outcome. The AI-based BOT will then also provide objective feedback to managers to help them improve their skills for providing feedback.
The outcome of performance management leads to decisions on ‘Developmental Journey(s)’ and those on ‘Compensation Management’.
The Decision of Developmental Journey(s):
Personal biases of managers and people guide the decisions of learning and development. Usually there is a significant gap between aspiration and capability on the part of individuals. This is compounded by the manager’s bias of a perceived match between aspiration and capability of individuals on the one hand, and a suitable opportunity on the other. With Artificial Intelligence, the assessment of capabilities, based on evidence of performance and that of potential, will be relatively better than the assessment made by managers based on pure perception. The mapping of opportunities, the desired ideal profile for the opportunity, and the skills and potential of individuals will be more objective than the present subjective system. Decisions made based on such patterns analyzed by AI will also be more objective and effective for both the business and for individuals.
The Decision of Compensation:
Compensation decisions are very complex. They take into consideration performance and, in a lot of cases, the potential of people. In some cases, it is also combined with the benchmark compensation in the industry for the role and hierarchical level. Ideally, it should also factor in the expectations of people, their market value, and fairness within the company, as well as peer group companies. While there are fairly sophisticated algorithms, usually these work for a category of people rather than for individuals. This creates some amount of corporate socialism within the category, leading at times to mediocrity. If we were to use AI to establish patterns of performance, potential, compensation benchmarks and individual market value, we will be able to determine individual compensation more accurately and fairly.
Thus, Artificial Intelligence can help in better decision-making on manpower planning, productivity management, optimal goal-setting and right recruiting decisions, leading to best-fit teams. Admittedly, putting together a right and appropriate team is difficult, but then, getting the best out of this team is even more difficult. The use of AI can help with better and objective decision-making on performance management, compensation management, and development, thus allowing organizations to leverage their human capital better and helping individuals to be appropriately rewarded, while developing and growing.
To sum up matters, Artificial Intelligence will change the face of the HR function and the quality of human capital. It will also help in harnessing this scarce capital significantly better than what is being done today.