Demystifying HR analytics: Descriptive to prescriptive and predictive analytics
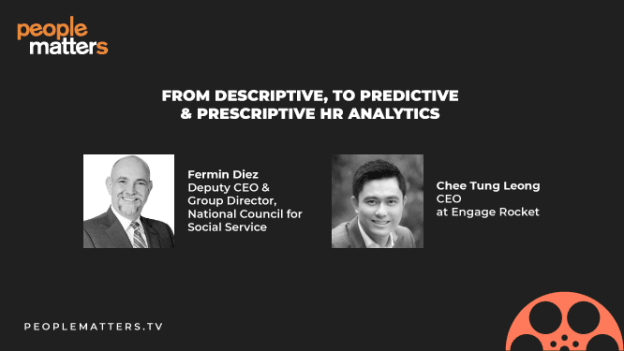
The shroud of mystery that has forever veiled the realm of HR analytics (much like any form of analytics) has been lifted with HR functions and processes becoming a lot more open, approachable and relatable lately. With more people understanding it, not only is there more stakeholder buy-in but there is also a larger group that is making the shift as HR analytics moves along its evolutionary course. Moreover, with AI making its presence felt and sought after, there is often the need to understand and plugin counter-intuitive AI generated predictions. Getting the whole organization onboard with these changes is crucial, albeit an arduous task.
Challenges to overcome
- Adept adaptation: Fermin Diez pointed out that the primary challenge has been that of adaptability since current HR professionals had entered the domain with a very different set of skills and strengths than the ones demanded presently. While the world has made its way onto AI (Artificial Intelligence) and a need for high data availability and HR has followed suit, there still needs to be a deeper adaptation and internalization of the changes.
- Guilty gut: There is often resistance to adopting AI since most professionals feel that their gut feelings and intuitions are enough to guide them through. “They often think on the lines of why do I need AI when I’ve been in recruitment for 20 years and I know whom to hire instinctively,” said Diez. To be able to distrust our gut might thus be a hard shift to make. He added that, “Our ability to predict a good hire is correct just a little less than 50 per cent of the time so we might as well flip a coin the next time we’re interviewing someone!” and stressed on the need to embrace predictive HR tools that are available today.
- End of empathy: Leong Chee Tung talked of being overly dependent on analytics and said, “On the flipside, when you are too analytical, you risk losing out on empathy”. He stated the case of a tech company that recently realized that one of their algorithms were routinely biased against women in hiring. Losing the human touch and not being able to strike the right balance between people and analytics is a serious challenge since at the end of the day, HR needs to be human in managing human resources.
The need of the hour (and of the recent future) is thus to be able to deal with data silos, to look at the human and experience side of HR as well and also to be able to plug in the right operational metrics into the right gaps at an everyday level. Chee Tung mentioned that, “The operational data gives you the what but you need to look at the experiential data to understand the why and then have a more holistic understanding.”
8 steps towards HR analytics
- Inserting the business variable: In order to understand the business implications of HR interventions, the need arises to incorporate the business variable (Y) into the picture. This would look at profits, revenue, market share and the (X) variable, being the HR variable, will look at the people aspect.
- Forming a hypothesis: The second step demands the creation of a hypothesis through the observation of the data. This hypothesis is essential since it could lead to the establishment of a cause-effect perspective.
- Procuring data: As in any objective statistical process, this step involves procuring the data that needs to be analyzed once the issue is located.
- Analysis: This is where the data is treated to answer the question posed by the hypothesis.
- Comprehension: To be able to devise ideas based on predictive analytics and to go beyond the statistics. This also involves being able to look at the answer to the hypothesis and debate on whether that is indeed the best possible answer.
- Building recommendations: In the third to last stage, the analysis of the data needs to be looked at in a way that can render the formulation of strategies that would be possible to implement. The strategies that promise results are thus the only ones that win recommendations.
- Visualization and crystallization: The recommendations made by the HR department are visualized first through tools like Tableau or the likes. This ability to visualize concepts makes the ideas more practical and realistic. The crystallization of these concepts is just another way to ensure the quality of the ideas visualized.
- Checking results: The results that are produced after all the steps are not the real results the business seeks. The results should be a matter to study to understand if the measures taken were effective or not. Repetitive studies like this can devise new ways to better the results.
These basic steps are the essence of analytical thinking and while the paraphernalia around them may change from one organization to the other, the heart of the matter remains constant and rooted in these stages. The implementation of this understanding is what would help businesses move from descriptive analysis that just enumerates “the what” towards predictive analysis that could open our eyes to the “what if” while also prescribing the right path ahead.
The road ahead
Leong Chee Tung said, “Instead of thinking in HR metrics we could think in business metrics.” With a strategy devised and ready to implement it is also vital to know where to implement it and the knowledge on business metrics that HR person needs to have. The crux of the matter thus still remains attaining a balance between HR and analytics to be able to truly utilize HR analytics in ways that promote positive change.
Being able to change in favor of HR analytics and to be able to make the most of its offerings, the HR stratum has to move as a whole. Change cannot be limited only to a few companies while the others carry on as onlookers. Being able to change at this moment will not make the companies game-changers because the game has already changed and we are all just playing catch-up. That said, we still must change to truly embrace analytics in the way it has been intended – as a multifaceted interface between people, processes and technology.
Diez talked of the shift towards becoming more inclined towards HR analytics and said, “I don’t think it’s about big departments, data science or big software. I think it’s about mindset.”
Are we ready to alter our mindsets? Are we already late in making the shift and should start proactively preparing for the next big thing? These are questions that would loom large over any change being it sudden or glacial and irrespective of whether we know the right answers (or whether there even are “right” answers to these questions), change we must anyway to embrace the path ahead.
The above article is based on excerpts of the PeopleMattersTV session on HR analytics.