A meaningful new approach to AI & Machine Learning
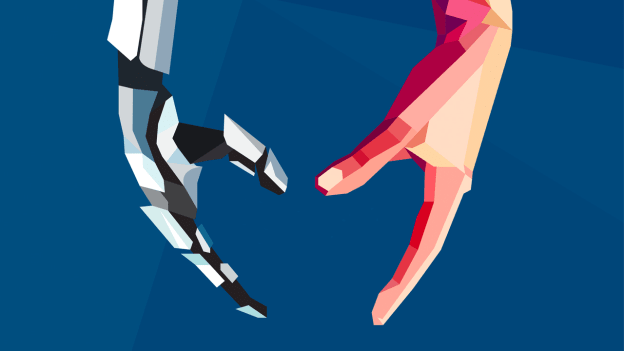
When a tech team sets out to solve a problem there is generally a hypothesis around what the problem is, what or who causes the problem and the result that should be delivered by the solution. The thing that is rarely considered is what specific, emotional impact that the tech will or should have on its users. Even less considered is that we, as people, present the greatest challenge for building effective human resources technology.
Human bias plays a significant role in the development of HR technology. My team and I over the course of 5 years built a technology to help solve this problem. Here’s a transparent view of what we did wrong, what we did right and how human emotion is and should be a driver of innovation.
The HR tech problem we tackled is ‘matching’. Matching people to jobs is a deceptively hard problem to solve because:
1) Companies, jobs, and candidates are very dynamic and
2) Both candidates and recruiters have biases that are inherent in us all
The likes of LinkedIn, Indeed, Zip Recruiter, Amazon and many other resource-rich companies have attempted to solve this problem with mixed results.
Over years of research and development and by learning from others who failed before us, we identified several key components to solving the ‘matching’ problem, whether it were developed for HR tech or another industry.
A new approach to AI & Machine Learning
In general terms, you can categorize machine learning as either ‘black box’ or unsupervised learning or as ‘white box’ which is commonly referred to as supervised learning.
A white box learns from past events and you can understand why it’s producing certain results and how variables are affecting it’s learning, resulting in far greater transparency. This is often referred to as linear or regression/decision tree models, which are linear in nature. The challenge with a white box strategy is that supervised learning also learns historical bias. In HR this means that it might learn that ‘men are better in technical jobs’ because men have historically been hired for technical roles more than women.
Black box models are harder to explain because users can only see the relationship between input and output but can’t see any of the underlying reasoning behind its decisions.
A human brain is a good example of a ‘black box’ of sorts. Other terms commonly used for this type of learning are deep-learning and random forest. This type of learning model is non-linear but much like a human brain, it can have a mind of its own. Black box results are more accurate in many other use cases but they are problematic in HR tech because they can also create a mind of their own and recruiting teams need to have some context to the data they are provided.
Our successful matching models come from what we call a ‘grey box’ approach, somewhat like a venn-diagram of multiple algorithms, with one very key difference, bias removal is it’s priority. Removal of bias is not a ‘nice to have’ objective, but rather an absolute, must-have constraint.
Human and ethical approach to AI
Because our first AI and machine learning models were built specifically for the HR Tech industry, we knew that it would be paramount to remove bias. Our white-box approach taught us how much bias had been baked into the job matching process for years. The bias was on the part of employers but also on the part of candidates. Both were causing a significant job to candidate matching challenges.
Common bias drivers:
1. Candidate names indicating gender
2. Recruiters think the candidate isn’t eligible to work in the US due to name only
3. First and last names causing ethnic bias
4. Photo of a candidate causing both positive and negative bias
5. Resume length, format, structure and design introduce enormous bias
Less understood bias drivers:
1. Employment gap
2. Job movement across industries
3. Job history outside of perceived ideal employers
4. Age as demonstrated by university graduation date
5. Transferable skills aren’t understood, readily seen in veteran hiring
Candidates also bring a great deal of bias to their reading of a job posting. These include confusing one company with another, not applying to a company that they have never heard of, and making company-wide judgments based on the negative actions of a small group of past employees.
Matching cannot be accurate without identifying key bias’ and developing technology to help humans remove this bias from the process. In HR tech bias removal is most important at the top of the funnel because without leveling the playing field at the beginning of the process it’s virtually impossible to deliver an unbiased slate of qualified candidates to interview.
Agnostic Solution Partnering
A key component of removing bias is removing bias from the data. The data has to be agnostic, diverse and structured.
1. The data can’t come from a certain company, certain job board or a certain industry.
2. Data needs to include as many different types of jobs and candidates as possible. For example, currently at Ai4Jobs powered by ThisWay we have built learning on 48,000 job types and 350 million people assisted by more than 3,000 global partners.
3. Unifying the structure of the data removes the bias that comes from letting well-structured data dominate less structured or ‘noisy’ data.
Lastly is a bias that we overlooked for years. It’s an accessibility bias and we are addressing this now.
Accessibility bias is building a business model, cost structure or data model that constrains access to the degree that only enterprise companies have access to the unbiased system. This also means that the only candidates that can receive fair matching are those that are interested in working for these enterprise companies, which flies in the face of the goal of removing bias.
Partnering towards an agnostic solution is made more difficult in incumbent organizations because they can’t afford to give their competitors an advantage. This is an instance where being an agile startup provides great benefit.
Emotions as a driver for innovation
Companies are so accustomed to using bias systems that many believe that qualified and diverse talent doesn’t exist. Candidates also believe they know all the companies that hire for their type of jobs in their location of choice.
When bias is removed the diversity of options is truly enlightening. Many times these candidates are already sitting inside the company applicant tracking system. But like gold nuggets in a mountain, the company needs a precision tool to help them mine the gold.
The best companies and recruiters are proactively seeking diverse talent because they know greater ROI that comes from this strategy. The success of the iPhone, Google Maps and many other technologies are closely tied to this user experience and resulting ‘delight’.
When people first experience unbiased matching results this often creates an emotional response. That happiness, or delight, is why we focus on building unbiased matching that matters.
Aligning tech objectives so that outcomes improve the lives of our fellow humans is the ultimate objective. Our view is that this is best accomplished when bias is diminished or removed.