Here’s how talent analytics is revolutionizing people strategy
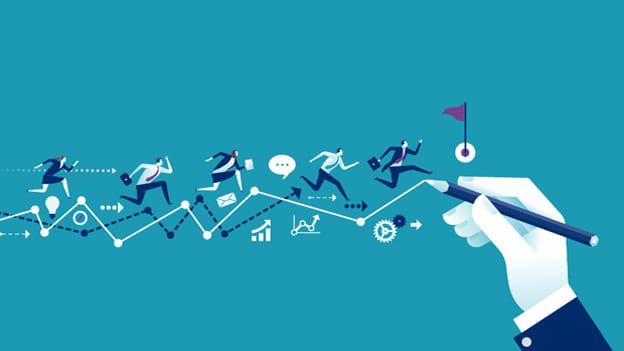
Top performing companies have been quick to identify talent as a critical competitive differentiator. No wonder, costs associated with talent management have evolved from being considered as a business expense to long-term strategic investment, bringing to the fore questions like:
- How to forecast the demand and supply of talent in short and medium term?
- How to attract and retain best-in-class talent?
- How to manage the ever-increasing total cost of workforce?
- How to keep a focus on the development of key talent?
- How to engage an increasingly diverse and globalized workforce?
- Determining an optimal workforce mix to deliver maximum value to stakeholders?
In order to answer these questions, the human resources (HR) function is rapidly learning not to rely on their gut feelings to determine their next decision impacting talent – whether it is to do with forecasting demand and supply of talent or identifying, recruiting, developing, and retaining the right people.
Combined new capabilities of human and machines put together are changing the way we work and with that the employer-employee relationship in a more fundamental way than ever before, driving organizations to adopt a more “scientific” approach to decision-making around people. Enter Talent Analytics and Workforce Planning! Though organizations understand the importance of talent analytics, many are grappling with even the most basic questions of where and how to start the process.
Now, it is not uncommon for companies and brands to use big data to improve customer experience and business. For example, online e-commerce business platforms analyzing shopping patterns and preferences to predict consumer behavior. It has truly revolutionized the way companies reach and target their customers.
Similarly, today organizations collect multiple data points on employees and use it to design and define people policies and decisions. An HR analytics maturity model such as the one illustrated in figure 1, can bridge the understanding of an organization’s current state and determining future vision in this area. This HR analytics maturity model, developed by Willis Towers Watson, has six levels of maturity outlining how an organization evolves from “Reactive Reporting” to “Predictive Analytics.” We have observed that the ROI on decisions taken on “Predictive Analytics” is exponentially higher than decisions taken using “Reactive Reporting.”
Predictive analytics, the science of using data and statistics to predict likely outcomes, is emerging as a high-interest area, yet only 3% organizations are currently leveraging it for making people decisions. Lack of skills to fully leverage the value of talent analytics is a big challenge faced by companies in India. Progressive companies are using predictive analytics to add tangible value to their stakeholders, employees, and management.
While creating a predictive analytics capability, it is very important that an organization first understand the key people-related challenges that the model is trying to solve. Often, companies want to run exploratory predictive analytics to assess whether there are any people related issues. However, organizations should first understand the business issue and then design a solution around it — rather than try to fit an existing solution into an unidentified problem.
A number of statistical techniques are available as part of HR analytics that helps organizations in not only anticipating attrition but also in designing effective retention strategies based on factors identified during this exercise. Here is an example of a step by step approach to the designing a retention model:
Segment the workforce – Workforce in an organization is not homogeneous, and a common retention programme might not be able to address the issue of attrition holistically. Therefore, clusters of employees with similar characteristics and reasons for leaving an organization need to be formed. This needs to be followed by a detailed analysis to understand the key factors influencing attrition within these clusters and then design retention strategies to target segments within the workforce.
Predicting the Probability of attrition at an employee level –The predictive risk score quantifies the probability of an individual leaving an organization in a specific time frame. This score can be to classify employees with high, medium, low risk of leaving the company. Equipped with knowledge of the attrition risk scores and the employee clusters, organizations can introduce a targeted intervention.
Predictive Model on Quality of Hire - Meet the New Age Recruiter
Companies are fast discovering that traditional methods have limited success in hiring high performers.
HR Analytics can help organizations reduce the risk of a bad quality hire by quantitatively bringing different candidate attributes into a single model and objectively scoring the probability of the success of an individual in the organization. This is done through modeling based on the profiles of successful employees in the organizations. Potential candidates are then evaluated on the same model, and a probability score of success is allocated to each candidate.
Thus, it is possible for the talent acquisition teams to have an objective selection criterion based on the probability of success of a potential hire for a particular role.
This is indeed a big step forward for HR in moving away from gut-based decisions to evidence-based decisions while selecting candidates.
In my experience of working with organizations across the Asia Pacific region, talent analytics and workforce planning need to be an integral part of business strategy and not something HR does in isolation.
I am optimistically looking forward to a time when companies leverage data and analytics to realize the full potential of their most important asset – employees.
Let’s unleash the power of people data.