Trump, Polling and Predictive Analytics
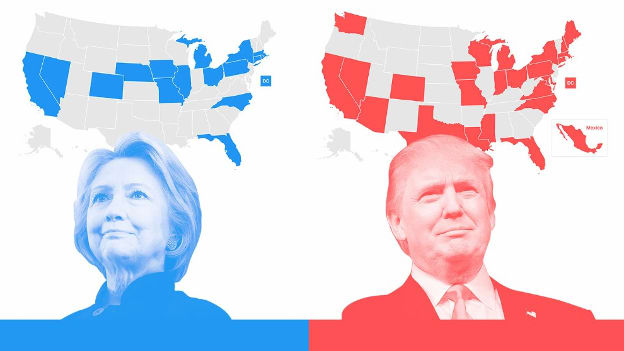
Earlier this week, the world believed that Donald Trump had only 10 to 15 percent chances of winning the US presidential elections. But pollsters and analysts were all in for a big shock last night. Despite many odds that were highlighted against for Trump in his race, the next US president is slated to be Donald Trump.
Of course polling for elections is a different ball game altogether than doing it for an organization, comparing polling for 200 million voting-age Americans is strikingly different from polling for a few thousand people in an organizational setting. But are such numbers relevant for corporates who try to understand underlying sentiments and intent of their teams when they analyze results of data collected from surveys and polls? Taking cue from the US presidential elections, we can safely state that the following were some of the things that the US polling experts got wrong, and how this can help us to reflect when we use surveys, polling and data analytics to predict results while taking into cognizance the sentiments of people:
People can have hidden intentions, unexpressed emotions and they can change their minds
A poll or a survey does not represent the truth nor a complete picture. People can change their minds, they can decide they don’t want to share their real opinions or they can just say what they think you want to hear. Analysts today are talking about “hidden Trump voters” or people who were embarrassed to admit (even anonymously) that they will vote for Trump because of social desirability bias. And they are also talking about the low levels of readiness of the American voters for a female president and how some may have not been able to actually admit that until reaching the voting booth. Such insights are hard to predict because eventually every individual’s opinion is personal and it is difficult to do mathematical modeling when inherent opinions and emotions are unpredictable.
Fitting prediction results into stakeholder’s expectations
Poll predictions during the US elections were selectively made in favor of the opinion of a particular segment of the population. The New York Times predicted 85 percent chance of victory for Hillary Clinton and The Huffington Post put Clinton’s chance to win at 98.3 percent. The Princeton Election Consortium's probability of a Hillary win was a ballsy 99 percent. It is believed that there was a potential confirmation bias to how the opinion polls were weighted.
Dangers with random sampling
While random sampling is used when polling and even when conducting surveys within the organization, many a times such samples are biased. Sampling can negate subgroups that have had different experiences and hold distinct opinions on the topic in hand. The randomized approach makes it a challenge to identify hidden pockets of dissatisfaction, and in some cases, this is where the problems can be.
In business, as much as in politics, we are obsessed with trying to predict the opinions, sentiments and emotions of our teams. The proof of the accuracy of such predictions will come from combining these, with a very strong ear at the ground that is listening to the underlying currents and sees them coming.